In recent years, the financial markets have witnessed a transformative shift with the advent and rise of algorithmic trading. Once the domain of institutional investors and hedge funds, these sophisticated trading strategies are now becoming increasingly accessible to the average investor.
This post delves into what algorithmic trading is, how it’s changing the investment landscape, and what it means for the everyday market participant.
What is Algorithmic Trading?
Definition: Algorithmic trading, commonly referred to as algo-trading, employs sophisticated computer programs and algorithmic systems to execute trades in financial markets. These algorithms are designed to identify trading opportunities based on market data, statistical analysis, and, often, complex mathematical models.
Unlike traditional trading, which involves human decision-making and manual execution, algo-trading automates the process, executing orders based on predefined criteria such as timing, price, quantity, and sometimes even the type of order. This automation enables executing trades with greater speed, precision, and efficiency than human traders can typically achieve, and they can take place over various time frames, from milliseconds to several days.
High-Frequency Trading (HFT)
A subset of algorithmic trading, HFT is characterized by extremely high speed and a high turnover rate of trades. It leverages advanced algorithms and high-speed data networks to execute orders in fractions of a second. HFT strategies are designed to capture small price discrepancies and arbitrage opportunities that exist for a very brief time period. Because of its speed and scale, HFT can significantly influence market liquidity and volatility.
Common Strategies in Algorithmic Trading
1. Arbitrage
This strategy seeks to exploit price differences of a similar asset across different markets or different forms of the same asset. For example, an algorithm may identify a price discrepancy between a stock listed on two different exchanges and quickly buy at the lower price and sell at the higher price, earning a profit from the difference.
2. Trend-Following or “Momentum”
These strategies involve algorithms that identify and follow established market trends. They use indicators like moving averages, price level movements, and channel breakouts to determine when to enter or exit trades. The focus here is on riding the momentum of market trends, rather than predicting reversals.
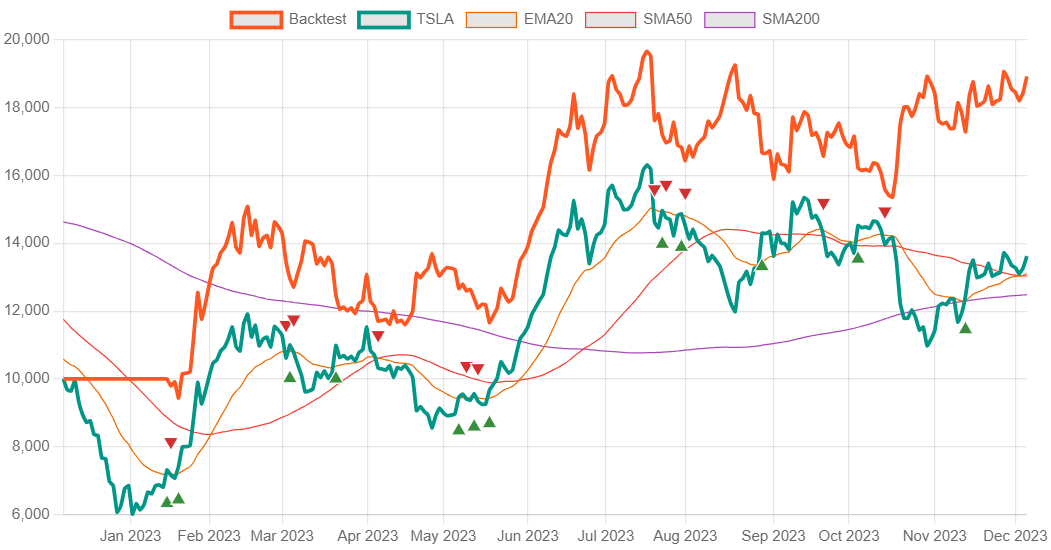
A number of these trend-following strategies (also known as Momentum algorithms) algorithms can be followed and backtested with Disfold DeepFinance, which will help you familiarize with algorithmic trading, evaluate risks and potential successful strategies, and benchmark and refine your own strategy to increase your investing and trading profits.
3. Market-Making
In this strategy, algorithms are used to provide liquidity to the market by continuously buying and selling securities and profiting from the spread between the buy and sell prices. Market-making algorithms place limit orders and adjust their pricing and quantity based on market conditions.
Historical Context and Evolution
Algorithmic trading began to take shape in the 1970s with the introduction of computerized trading systems. Initially, these systems were rudimentary, often limited to basic arithmetic calculations and data analysis.
The establishment of the NASDAQ in 1971 marked a significant milestone, introducing electronic trading and setting the stage for algorithmic strategies.
Large institutional investors and proprietary trading firms were among the first to adopt these computer-driven trading methods. They used algorithms primarily for efficient order execution and managing large trades to minimize market impact.
Technological Advances
The explosion of computing power in the 1980s and 1990s enabled more complex algorithms and faster execution. Traders began to leverage mathematical models and statistical analysis to identify trading opportunities.
With the advent of big data and machine learning, algorithmic trading evolved to include predictive analytics, allowing traders to anticipate market movements based on historical data patterns.
Improved internet connectivity and the rise of electronic trading platforms in the late 1990s and early 2000s facilitated a more connected global market, allowing for real-time data analysis and immediate execution of trades.
Regulatory Landscape
In the early years, algorithmic trading faced minimal regulatory oversight, as the rapid evolution of technology outpaced regulatory frameworks.
Significant market events, such as the 2008 financial crisis and the 2010 Flash Crash, prompted regulators to scrutinize algorithmic trading practices more closely.
Regulatory bodies like the SEC in the U.S. and FCA in the UK have implemented measures to enhance market transparency and stability. These include requirements for more detailed reporting, risk management controls, and measures to prevent market abuse and manipulation.
Impact of Algorithmic Trading on the Market
Market Efficiency
Price Discovery: Algorithmic trading contributes to more efficient price discovery by rapidly assimilating and acting upon available market information. The speed and volume at which algorithms can process market data mean prices reflect new information quicker than they would through traditional manual trading.
Liquidity Enhancement: By continuously placing buy and sell orders, algorithmic trading contributes to increased market liquidity. This makes it easier for other market participants to execute their trades at competitive prices without causing significant price movements.
Reduced Spreads: The increased liquidity and efficiency often lead to narrower bid-ask spreads, reducing transaction costs for all market participants.
Volatility
High-Frequency Trading (HFT) and Volatility: While HFT can enhance liquidity and efficiency, there are concerns about its contribution to short-term volatility. The rapid trading strategies of HFT can amplify price movements, particularly in turbulent market conditions, as seen in events like the 2010 Flash Crash.
Feedback Loops: Automated algorithms may sometimes create feedback loops. If many algorithms respond similarly to certain market conditions, it can lead to exaggerated market movements, both upward and downward.
Mitigating Measures: To address these concerns, financial regulators and exchanges have implemented measures like circuit breakers and volatility halts, which temporarily stop trading in a stock or market to prevent extreme volatility.
Accessibility for Retail Investors
Technological Democratization: Recent technological advancements, especially in cloud computing and software-as-a-service (SaaS) models, have made sophisticated trading algorithms more accessible to retail investors.
One such platform is Interactive Brokers which provides a programmatic API to query data and place different trading orders. Remark that you can earn up to $1,000 of free IBKR Stock by opening an account on Interactive Brokers with my link! (You’re welcome!😊)
Benefits of Algorithmic Trading
Efficiency and Speed: Algorithms can process vast amounts of data and execute orders far more quickly than humans. This speed allows for taking advantage of market opportunities almost instantaneously.
Removing Emotional Decision-Making: One of the key advantages of algo-trading is its ability to eliminate the emotional biases inherent in human trading. Algorithms follow predefined rules, making decisions based on data and analysis, not on fear, greed, or other emotions that can lead to irrational trading decisions.
Increased Precision and Reduced Errors: Algorithmic trading is highly precise, adhering strictly to the parameters set by the trader. This precision reduces the likelihood of errors, which are more common in manual trading.
Backtesting Capabilities: Traders can use historical data to backtest trading strategies before implementing them in live markets. This helps in identifying and refining effective strategies and reducing potential risks.
Cost-Effectiveness: By executing trades at optimal times and minimizing the impact of large trades, algorithmic trading can reduce transaction costs. This is particularly beneficial for strategies involving a large number of trades.
Risks of Algorithmic Trading
Over-Reliance on Technology: Heavy reliance on algorithms can lead to complacency. Traders might overlook the need for continual oversight and intervention, especially in volatile or abnormal market conditions.
Systemic Risks and Technical Failures: If many market participants are using similar algorithms, it can create systemic risks. Moreover, technical failures, such as connectivity issues or software glitches, can result in unexpected losses.
Algorithmic Complexity and Misinterpretation: Some algorithms are highly complex, and a lack of understanding can lead to unintended trading actions. Misinterpretation of market conditions by the algorithm could result in significant losses.
Market Vulnerability to Manipulation: There are concerns that algorithmic trading can be used for manipulative strategies like quote stuffing or layering, which can distort prices and market liquidity.
Regulatory and Compliance Risks: As regulations evolve, there’s a risk that an algorithm might inadvertently violate trading rules, leading to legal and financial repercussions.
Getting Started with Algo-Trading
User-Friendly Platforms: Many fintech companies now offer user-friendly platforms that allow retail investors to use or customize algorithmic trading strategies without needing a background in coding or high-level finance.
Educational Resources: The proliferation of online educational resources and communities around algorithmic trading has also empowered retail investors to understand and leverage these tools for their investment strategies.
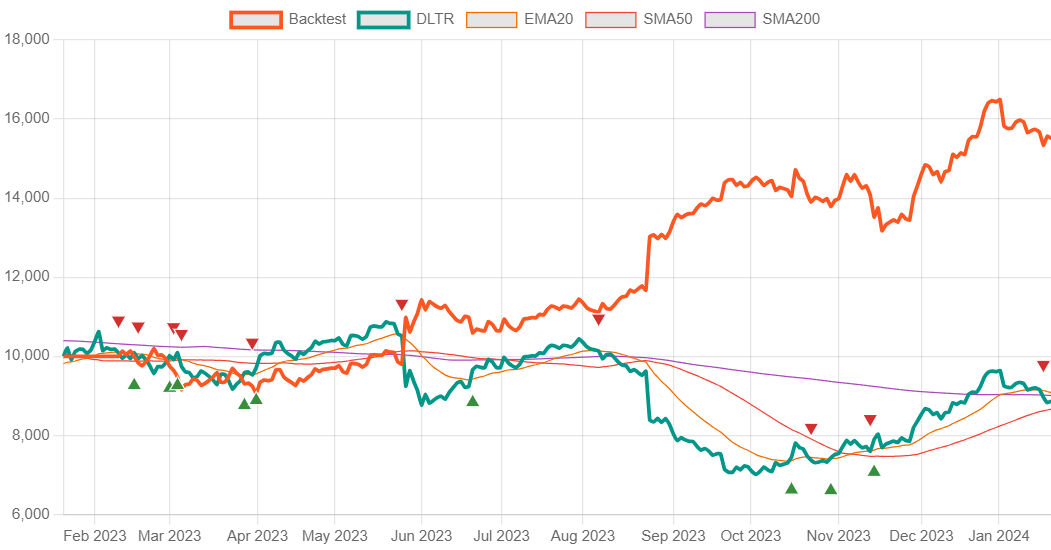
One such platform is Disfold DeepFinance, which will help you familiarize with simple trend-following algorithmic trading on the daily timeframe, assess risks and potential returns, with our very user-friendly, convenient and affordable platform.
If you are interested you can then follow any number of live backtests, allowing to simply see how the algorithm would trade such or such of your favorite stocks, and understand what benefits and risks are at stake. To get started, just subscribe to Disfold DeepFinance, and you will get a head-start in your journey towards a deeper understanding of the market and taking your investing and trading to the next level.
Algorithmic trading represents a significant shift in how markets operate and how individuals can participate in them. As technology continues to advance, it’s becoming an increasingly important tool for investors of all types. While it brings new opportunities, it’s crucial for investors to educate themselves and approach this new landscape thoughtfully and strategically.
Well written Sir. This article is truly useful to me.